Predictive Maintenance For Mobile Assets Within The Mining Industry
Introduction
The mining industry relies heavily on mobile assets, such as haul trucks, loaders, and drilling rigs, which are crucial for the extraction and transportation of materials. The operational efficiency of these mobile assets directly impacts productivity and cost management. Mobile equipment in mining operations are subject to demanding conditions and intensive use, leading to deterioration that can culminate in unanticipated equipment failures.
The Challenge
Mobile assets in mining operations face extreme conditions and heavy usage, leading to wear and tear that can cause unexpected breakdowns. Challenges include the following:
- Harsh Working Environments: Continual exposure to abrasive materials, vibrations, and heavy loads accelerates equipment degradation.
- Unpredictable Maintenance Needs: Predicting maintenance needs for a diverse fleet of mobile assets with different operating conditions and lifecycles is complex.
- Scheduling and Downtime: Strategically scheduling maintenance to minimize downtime and operational disruption is a persistent operational challenge.
- Diverse Fleet Complications: A wide variety of mobile assets with different ages, technologies, and maintenance requirements complicate standardization of maintenance procedures.
- Data Overload: The vast amount of data generated by modern mobile assets can be overwhelming, making it difficult to extract actionable insights for maintenance.
- Integration of New Technologies: As fleets modernize, integrating new technologies with existing assets for streamlined maintenance can be a hurdle.
- Compliance and Safety: Keeping up with stringent industry regulations and ensuring the safety of operators is always a primary concern, particularly when maintenance lapses can lead to critical failures.
- Resource Allocation: Deciding how best to allocate limited resources, including personnel and spare parts, can be a significant challenge, especially in remote mining operations with limited access.
Addressing these challenges is essential for optimizing the lifecycle of mobile assets and maintaining the high productivity and safety standards required in the mining industry.
The Solution: XMPro for Predictive Maintenance of Mobile Assets in Mining
XMPro’s Intelligent Business Operations Suite (iBOS) is adeptly tailored for the nuanced challenges of maintaining mobile mining assets, ensuring operational excellence through comprehensive predictive maintenance capabilities. The solution integrates advanced sensors, real-time data processing, and predictive analytics to enhance the efficiency and reliability of mobile assets. With detailed visualizations of asset locations and statuses, operators can quickly identify and address issues. Real-time monitoring provides alerts for critical conditions such as engine health, hydraulic fluid quality, and tire pressure, allowing for timely interventions. The system’s recommendations and work order management streamline maintenance activities, mobilizing crews, scheduling repairs, and managing spare parts inventory. Configurable dashboards and reporting features support in-depth analysis and regulatory compliance, ultimately enhancing operational efficiency and asset longevity. This comprehensive, data-driven approach ensures that mobile mining assets are maintained at peak performance, reducing downtime and optimizing resource allocation.
Key Features
Integrated Real-Time Asset Monitoring: XMPro iBOS unifies sensor data from across mobile assets, providing a real-time overview of mechanical health, from engine performance to structural integrity, critical for preemptive maintenance actions.
Advanced Predictive Analytics: The system employs advanced algorithms to predictively assess the condition of mobile assets, offering foresight into potential failures and enabling timely, cost-effective interventions.
Dynamic Digital Twin Capabilities: XMPro iBOS generates dynamic digital twins of mobile assets, simulating real-world conditions for detailed scenario analysis and maintenance planning.
Automated Scheduling for Optimal Maintenance: Predictive analytics drive the automated scheduling feature, ensuring maintenance is executed without disrupting the mining workflow.
Configurable Dashboards for Decision Support: Operators receive actionable insights through adaptable dashboards, enhancing visibility into asset performance and health, which supports strategic decision-making.
Through XMPro iBOS, mining companies gain a predictive maintenance solution that minimizes downtime, maximizes asset lifespan, and ensures ongoing safety and compliance.
How XMPro iBOS Modules Work Together To Create This Predictive Maintenance Solution
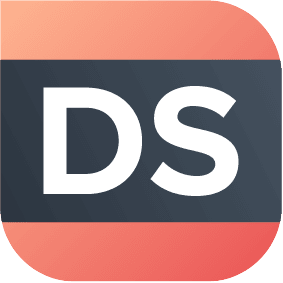
XMPro Data Stream Designer
XMPRO’s Data Stream Designer lets you visually design the data flow and orchestration for your real-time applications. Our drag & drop connectors make it easy to bring in real-time data from a variety of sources, add contextual data from systems like EAM, apply native and third-party analytics and initiate actions based on events in your data.
Figure 1. Haul Truck Predictive Maintenance – Engine Health Data Stream
This predictive maintenance data stream reads haul truck telemetry data and CAT Minestar error data, merges them, and broadcasts the combined data for analysis. It performs predictive failure modeling and anomaly detection for engine health, calculates immediate critical thresholds, and joins operational data for context. The results are filtered to remove false positives and generate actionable maintenance recommendations. These recommendations are then published to an interactive visualization platform, integrated with XMPro’s app and Unity visualization, and further enhanced with insights from Azure OpenAI for comprehensive engine health monitoring and proactive maintenance actions.
Figure 2: Haul Truck Idle Time & Performance Monitoring Data Stream
This haul truck performance monitoring data stream ingests real-time telemetry data on power output, payload weight, and fuel consumption. The data is merged and broadcasted to subsequent processes. It is then contextualized with work order and maintenance data to provide a comprehensive view of operations. The stream calculates key performance metrics, including idle time, running time, and downtime. The integrated data is analyzed to generate actionable recommendations, which are broadcasted to the XMPro app for visualization and interactive monitoring. This ensures optimal haul truck performance, reducing idle time and enhancing operational efficiency.
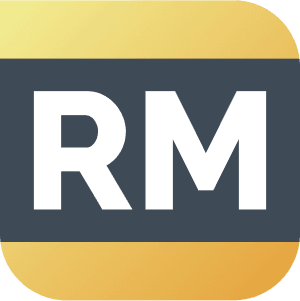
Recommendation Manager
XMPRO Recommendations are advanced event alerts that combine alerts, actions, and monitoring. You can create recommendations based on business rules and AI logic to recommend the best next actions to take when a certain event happens. You can also monitor the actions against the outcomes they create to continuously improve your decision-making.
Figure 1. Haul Truck Engine Health Low Recommendation
This haul truck engine health recommendation identifies a critical issue where the engine health is low, indicating an imminent risk of failure. It provides detailed event data, including idle times, tire back left inner pressure, tire back right inner pressure, and hydraulic fluid quality. Users can view and add notes, mark the recommendation as resolved or a false positive, and create a work request with special instructions. This recommendation ensures immediate attention to critical engine health issues, facilitating timely maintenance actions to prevent operational disruptions.
Figure 2. Configure With Granular Rule Logic
This engine health alert configuration allows users to set up granular rule logic for monitoring the engine health of haul trucks. The interface enables selecting the engine health metric and setting a specific threshold (below 80%) to trigger alerts. Users can categorize recommendations under “Mobile Assets,” enable execution order, and specify additional recommendation management columns. The configuration also allows the selection of a form, such as a work request, to be automatically enabled when the alert is triggered. Users can choose between manual or automatic resolution options, ensuring critical engine health issues are promptly and appropriately addressed to maintain haul truck performance.
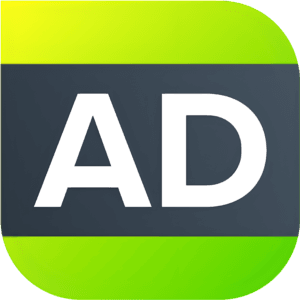
XMPro App Designer
The XMPro App Designer is a no code event intelligence application development platform. It enables Subject Matter Experts (SMEs) to create and deploy real-time intelligent digital twins without programming. This means that SMEs can build apps in days or weeks without further overloading IT, enabling your organization to accelerate and scale your digital transformation.
Figure 1. Mobile Asset Overview Dashboard for the Mining Industry Overview
This comprehensive dashboard empowers mining industry professionals to effectively monitor and manage a fleet of mobile assets spread across mining operations. With a detailed and dynamic interface, it gives a broad view of asset locations and statuses, enabling rapid decision-making to optimize fleet performance and maintenance.
Key Features
- Geo-Location Tracking: Showcases the real-time location of all mobile assets on a map, simplifying the tracking of equipment across expansive mining sites.
- Health and Maintenance Alerts: Integrates sensor data to deliver immediate alerts on asset health, highlighting those needing maintenance to prevent operational disruptions.
- XMPro Co-Pilot Integration: Leverages the smart capabilities of XMPro Co-Pilot to provide predictive insights and recommendations, enhancing asset utilization.
- Detailed Asset Performance Data: Offers in-depth analysis for each mobile asset, including usage statistics and operational efficiency metrics, supporting precise maintenance scheduling.
- Proactive Maintenance Scheduling: Utilizes predictive analytics to foresee and schedule maintenance tasks, ensuring assets are serviced without affecting mining activities.
- Centralized Oversight: Consolidates information for fleet-wide tracking, allowing for a quick assessment of overall asset status and maintenance needs.
Benefits
With this Mobile Asset Overview Dashboard, mining companies gain enhanced control over their mobile assets, leading to improved maintenance planning, increased operational efficiency, and extended asset life spans. It supports informed decision-making for resource allocation and ensures that assets are always ready to meet the demands of mining operations.
Figure 2. Asset Drilldown View – Mobile Assets in Mining Operations Asset Class Drilldown View – Mobile Mining Units
This powerful dashboard delivers detailed analytics on mobile mining assets, categorized by asset types such as dozers and haul trucks. Designed for versatility, it provides critical insights across the entire mobile fleet.
Key Features:
Alerts Overview
Classifying open alerts by criticality, the dashboard enables prioritization of maintenance actions for assets showing high-severity alerts, ensuring immediate attention to critical areas and preventing operational halts.
Work Order Status
The work order status pie chart offers a snapshot of asset availability and maintenance scheduling, crucial for minimizing downtime and planning resources effectively across the mobile fleet.
Performance Metrics (Last 30 Days)
Key performance metrics over the last 30 days highlight maintenance efficiency, showcasing the team’s effectiveness in addressing alerts and resolving issues, ensuring the mobile assets’ optimal performance.
Asset Filtering and Service Information
An advanced filtering feature displays upcoming service schedules, supporting a proactive approach to asset maintenance and operational readiness.
Recent Recommendations
Actionable recommendations for each asset are listed, equipping maintenance teams with precise, data-driven directives for immediate and future interventions, tailored to address specific asset needs.
Assets Overview
Offering a comprehensive listing of each mobile asset along with its criticality and risk score, the dashboard centralizes data for streamlined oversight of the mobile mining unit’s health.
XMPro Co-Pilot Integration
Incorporating AI-driven insights, XMPro Co-Pilot enhances decision-making with intelligent advice based on real-time asset data, augmenting the maintenance strategy for each asset type.
Navigation and Total Assets
Intuitive navigation tools indicate the scope of assets managed, with a total count provided, reflecting the dashboard’s extensive coverage across the mining operation’s mobile asset portfolio.
This dashboard becomes an indispensable tool for mining operations, driving improved maintenance outcomes, ensuring asset availability, and optimizing fleet performance.
Figure 3: Asset Analysis View – Mobile Mining Equipment Health
This Asset Analysis View is integral for monitoring the condition of mobile mining equipment such as haul trucks. The system emphasizes key performance indicators and health metrics tailored to the mining context, including engine status, load capacity, and operational efficiency. Utilizing predictive analytics, the dashboard calculates the Remaining Useful Life (RUL) of vehicle components, providing actionable insights for maintenance schedules. It enables operators to pre-emptively address potential issues, ensuring equipment reliability and reducing unscheduled downtime.
Key Features:
Interactive Equipment Models
Offering interactive visualizations, the dashboard allows for a detailed examination of equipment, identifying areas prone to wear or imminent failure. Users can interact with both 2D schematics and 3D models, focusing on critical subsystems such as engines, hydraulics, and drivetrains.
Error Identification and Preventative Actions
Intelligent diagnostics on the platform detect anomalies and provide maintenance alerts. For instance, it might flag “Hydraulic Pressure Drop” or “Engine Temperature Rise” as potential issues, along with recommended preventative measures to avoid costly repairs and operational delays.
Detailed Equipment Profiles
For each piece of equipment, the dashboard presents a comprehensive profile including type, model, service history, and hours of operation. This data forms the basis for informed maintenance planning and operational decision-making.
XMPro Co-Pilot Integration
The integration of XMPro Co-Pilot applies AI to analyze historical and real-time data, proposing specific maintenance actions. This AI support enhances the predictive maintenance strategy, helping to maintain high availability and performance of mining assets.
Maintenance and Service Tracking
The platform tracks and records all maintenance activities, documenting actions taken and their outcomes. This logging ensures accountability and contributes to the continuous optimization of asset performance.
By combining real-time monitoring with advanced analytics, the Asset Analysis View helps mining operations maintain the health and efficiency of their mobile assets, supporting sustained productivity and safety standards.
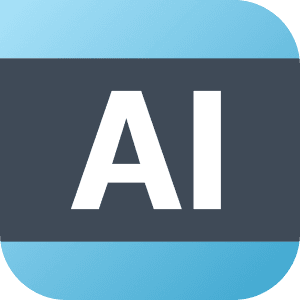
XMPro AI
Experience the transformative power of XMPro’s Intelligent Business Operations Suite (iBOS) – Featuring comprehensive AI capabilities, XMPro iBOS helps to significantly increase product yield, drastically reduce downtime, and ultimately eliminate unexpected business events.
Figure 1: Haul Truck Predictive Maintenance – Engine Health Data Stream
Embedding XMPro AI Agents in XMPro Data Streams enables executable AI and machine learning for algorithmic business processes, significantly enhancing the capabilities of operational digital twins. This integration allows for advanced features such as real-time analytics, MLOps, and seamless embedding of AI into core business processes.
In this example of haul truck predictive maintenance for engine health, XMPro’s AI Agents empower the data stream to accurately identify and predict potential engine health issues. The process begins with the ingestion of real-time telemetry data from haul trucks, which is then combined with error code data to provide a comprehensive view of the truck’s operational status. Machine learning models, including predictive failure models and anomaly detection, are applied to this data to detect deviations and forecast potential failures.
Embedded AI Agents
XMPro offers a variety of AI agents to support diverse operational needs, including:
- Azure OpenAI: Enhances natural language processing capabilities.
- OpenAI Assistant: Facilitates conversational AI integrations.
- Anomaly Detection: Identifies unusual patterns in data to prevent operational failures.
- Forecasting: Predicts future trends based on historical data.
- Kmeans Clustering: Groups similar data points for more effective analysis.
- MLflow: Manages the machine learning lifecycle, including experimentation, reproducibility, and deployment.
- Regression: Provides predictive analytics to understand relationships between variables.
By embedding these powerful AI agents, XMPro transforms AI models into valuable assets that drive business growth and efficiency, bridging the gap between data flow and operational AI.
Why XMPro iBOS for Mining Plant Operations?
XMPro’s Intelligent Business Operations Suite (iBOS) is expertly devised for the intricate challenges faced in the predictive maintenance of mobile assets within the mining industry. Here’s the transformation it brings:
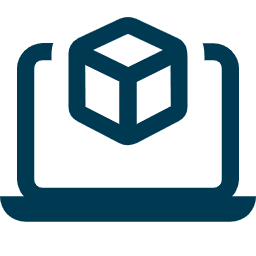
Advanced Intelligent Digital Twin Modeling:
XMPro iBOS constructs sophisticated digital twins, reflecting the detailed operations of mining equipment. It allows comprehensive performance analysis under varied conditions, vital for operational optimization.
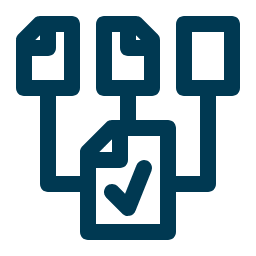
Advanced Sensor Data Integration & Transformation:
Real-time sensor data across mobile assets offer critical insights into performance metrics like vibration, load capacity, and engine status, which are essential for detecting early signs of potential failures and maintenance needs.
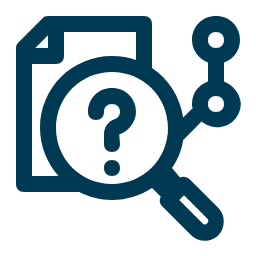
Predictive Analytics for Performance Enhancement:
Utilizing advanced analytics, XMPro iBOS predicts potential asset failures, enhancing operational parameters and enabling preventive adjustments, thereby ensuring continuous mining operations with minimized downtimes.
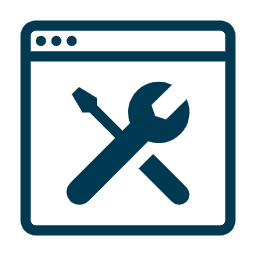
Maintenance Scheduling Optimization:
Performance data drives XMPro iBOS’s maintenance scheduling, transforming the approach from reactive to proactive, optimizing the maintenance cycle for various assets, and significantly reducing breakdowns.
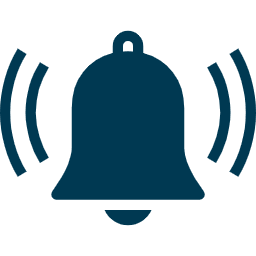
Real-Time Monitoring and Predictive Alerting:
Real-time monitoring and predictive alerting are critical components of XMPro’s iBOS for managing mobile assets within the mining industry. This ensures each mobile asset, from haul trucks to dozers, functions within the optimal parameters, thus enhancing efficiency and reducing reliance on manual intervention.
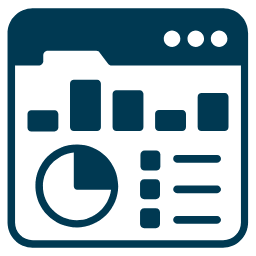
Configurable and Interactive Dashboards:
XMPro provides configurable dashboards that offer real-time insights into the health and performance of equipment across all dairy processing plants. These dashboards are designed to be interactive, enabling detailed scrutiny of specific operational aspects and supporting centralized management decisions.
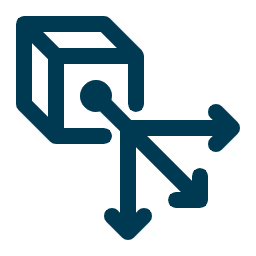
Scalability and Flexibility – Start Small, Scale Fast:
Designed to accommodate dairy operations of any scale, XMPro’s modular architecture allows for seamless integration and adaptability. This scalability ensures that mining plants can efficiently manage operations as they expand or adapt to changing market demands.
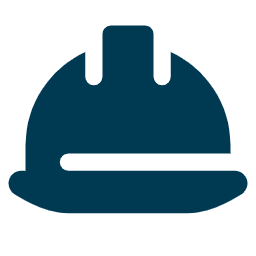
Enhanced Safety & Operational Efficiency:
XMPro boosts operational safety by identifying potential hazards and inefficiencies in the processing line, ensuring that all equipment operates within safe and optimal parameters. This contributes to a safer working environment and more efficient production processes.
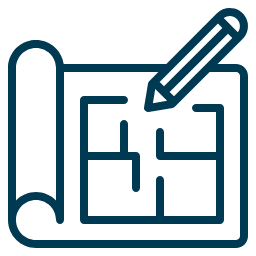
XMPro Blueprints – Quick Time to Value:
Offering quick time-to-value, XMPro Blueprints facilitate rapid deployment of intelligent operations solutions across mining operations. These templates are built on industry best practices, ensuring that plants can quickly realize the benefits of digital transformation.
XMPro iBOS caters to the predictive maintenance needs of the mining industry’s mobile assets with a suite that promises comprehensive, predictive, and integrated solutions, driving efficiency and safety across operations.
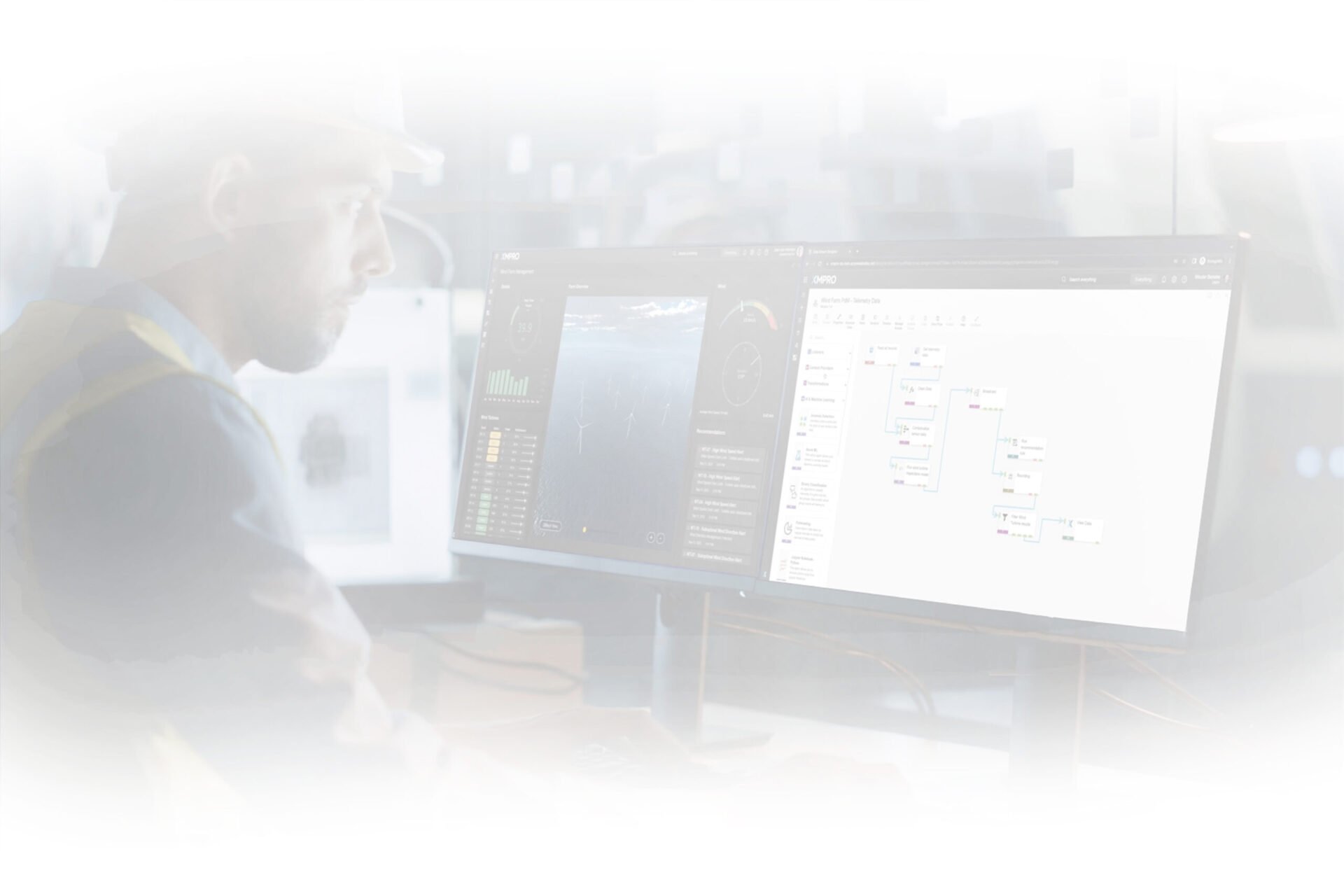