Bogie Health Monitoring in the Rail Industry
Introduction
Maintaining the health of train bogies is crucial for ensuring the safety and efficiency of rail operations. XMPro’s Bogie Health Monitoring Solution employs advanced technologies to detect early signs of wear or failure, aiming to enhance safety and minimize unscheduled repairs.
The Challenge
Rail operators face several challenges in bogie maintenance:
- Early Wear and Failure Detection: Identifying early signs of wear or failure in bogie components is essential to prevent accidents and ensure smooth operations.
- Maintenance Scheduling: Determining the optimal frequency for maintenance activities to maximize safety and minimize disruptions.
- Unscheduled Repairs: Reducing the occurrence of unscheduled repairs that can lead to operational delays and increased costs.
The Solution: XMPro iBOS For Bogie Health Condition Monitoring In The Rail Industry
XMPro’s solution for bogie health condition monitoring leverages advanced sensors and analytics to ensure rail safety and performance. It continuously monitors key bogie parameters like wheel wear, axle load, temperature, and vibration. Using machine learning algorithms, the system predicts issues before they escalate, providing real-time alerts for maintenance teams.
Configurable dashboards display critical data such as spring compression, bearing temperature, and vibration amplitudes. Predictive insights optimize maintenance schedules based on actual conditions rather than fixed intervals. An asset overview map shows the status of trains, crossings, and lines, with real-time recommendations for immediate attention. This technology enhances safety, operational efficiency, and the lifespan of bogie components.
Key Features
- Advanced Bogie Monitoring: Continuous monitoring of wheel wear, axle load, temperature, and vibration using state-of-the-art sensors.
- Data Integration and Predictive Analytics: Integrates sensor data and applies machine learning algorithms to predict potential bogie issues before they escalate.
- Real-Time Monitoring and Alerts: Provides real-time alerts to maintenance teams for any detected anomalies requiring immediate attention.
- Maintenance Optimization: Utilizes predictive insights to optimize maintenance schedules, focusing on condition-based interventions rather than fixed intervals.
- Customizable Dashboards and Reporting: Offers customizable dashboards displaying key bogie health data, alongside comprehensive reporting features for maintenance planning and regulatory compliance.
How XMPro iBOS Modules Work Together To Create This Condition Monitoring Solution
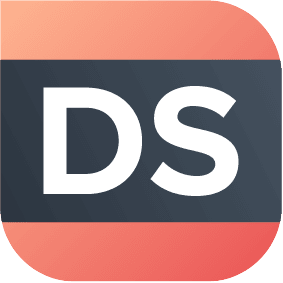
XMPro Data Stream Designer
XMPRO’s Data Stream Designer lets you visually design the data flow and orchestration for your real-time applications. Our drag & drop connectors make it easy to bring in real-time data from a variety of sources, add contextual data from systems like EAM, apply native and third-party analytics and initiate actions based on events in your data.
Figure 1: Bogie Health Condition Monitoring Data Stream
This bogie health condition monitoring data stream ingests real-time data from sensors measuring wheel vibration, bearing vibration, bearing temperature, and spring compression. The data is combined with historical work orders and downtime data, processed, and broadcasted for analysis. Critical thresholds are checked to flag high-risk conditions, generating alerts for maintenance teams.
An anomaly detection model identifies subtle issues, while a Remaining Useful Life (RUL) model predicts bogie component longevity. The data stream produces recommendation alerts based on these analyses. All alerts and recommendations are displayed in the XMPro App, providing actionable insights for timely maintenance and ensuring rail system reliability and safety.
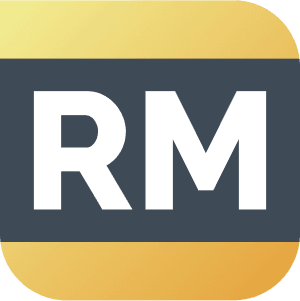
Recommendation Manager
XMPRO Recommendations are advanced event alerts that combine alerts, actions, and monitoring. You can create recommendations based on business rules and AI logic to recommend the best next actions to take when a certain event happens. You can also monitor the actions against the outcomes they create to continuously improve your decision-making.
Figure 1: Example Recommendation: Bogie Health Threshold
This bogie health threshold recommendation identifies an issue with the bogie exceeding predefined safety limits. It provides event data, including wheel vibration, bearing vibration, bearing temperature, spring compression, timestamp, and bogie ID. Users can add notes, mark the recommendation as solved or a false positive, and create a work request with special instructions if necessary. This recommendation helps ensure timely maintenance actions to address the issue and maintain safe railway operations.
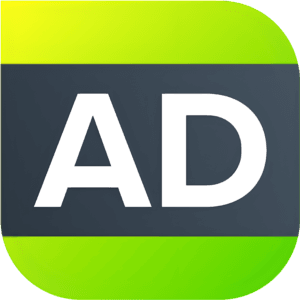
XMPro App Designer
The XMPro App Designer is a no code event intelligence application development platform. It enables Subject Matter Experts (SMEs) to create and deploy real-time intelligent digital twins without programming. This means that SMEs can build apps in days or weeks without further overloading IT, enabling your organization to accelerate and scale your digital transformation.
Figure 1. Real-Time Rail Asset Overview Dashboard
This dashboard provides a real-time view of rail assets with an interactive map that updates GPS coordinates of trains and track issues, offering a clear visual of railway lines. Each asset is marked with a color-coded status icon indicating its operational state, including alerts and error messages. The dashboard shows the overall status of asset categories like trains, crossings, tracks, maintenance vehicles, and substations, and highlights active recommendations from the system’s rule logic, such as exceeded bogie wear thresholds.
Key Features:
- Real-Time Asset Locations:
- An interactive map displays real-time GPS locations of rail assets, including trains, crossings, lines, maintenance vehicles, and substations.
- Color-Coded Status Icons:
- Assets on the map are marked with color-coded icons indicating their current operational status. Green signifies active and operational, yellow indicates warnings, and red represents critical issues needing immediate attention.
- Overall Asset Status Summary:
- A summary at the bottom provides a quick overview of the total number of active and inactive assets across various categories, including trains, crossings, lines, maintenance vehicles, and substations.
- Recommendations Panel:
- A list of active recommendations generated by the system’s rule logic, such as exceeded wheel wear thresholds, voltage fluctuations, and bogie health thresholds, ensuring immediate visibility of critical issues.
- Equipment Requiring Maintenance:
- A detailed graph and list show maintenance requirements across different assets, prioritizing those based on their upcoming service needs. This facilitates efficient maintenance scheduling and ensures timely interventions.
- Drill-Down Capabilities:
- Each section of the dashboard allows users to drill down into specific asset and recommendation details, providing granular insights and enabling targeted actions based on the system’s recommendations.
- Alerts and Error Messages:
- Real-time alerts and error messages are displayed, notifying maintenance teams of any detected anomalies that require immediate attention.
- Interactive Map Controls:
- Users can interact with the map to zoom in/out and click on specific assets to get more detailed information about their status and any ongoing issues.
This comprehensive view ensures that users have all the necessary information at their fingertips to monitor and manage rail assets efficiently, respond quickly to issues, and maintain optimal operational performance.
Figure 2. Asset Class Drilldown View
The Asset Class Drilldown view provides an in-depth analysis of rail track lines, presenting a comprehensive overview of current track alerts and work order statuses. It features a bar chart displaying the number of track lines with open alerts categorized by severity (high, medium, and no alerts) and a pie chart summarizing the status of current work orders (available, planning, and waiting). The asset list below details individual track lines, showing their last service dates, recommendations, and upcoming service schedules, allowing users to prioritize maintenance needs efficiently. Key metrics for the past 30 days, including new alerts, work requests, work orders, and time to resolution, are displayed for performance tracking.
Key Features:
- Alerts Overview: Graphical representation of open alerts categorized by severity – no alerts, medium, and high severity.
- Work Order Status: Displays current status of each asset, categorized as available, in planning, or waiting.
- Performance Metrics (Last 30 Days): Summarizes key metrics such as new alerts, number of work orders, open work orders, and open work requests. It also tracks the duration from alert initiation to work order completion, comparing it with the previous 30-day period.
- Asset Filtering and Service Information: Enables filtering across all train assets, showing details like the last service date, upcoming service schedules, and due dates.
- Recent Recommendations: Lists recent recommendations triggered for train assets, with options for users to view details and take necessary actions.
- XMPro Co-Pilot Integration: Features an interactive block where users can query the AI model, trained on internal data like train engine manuals, for specific advice on errors, warnings, and issues. This information can be directly linked to work order requests and triage instructions.
This dashboard is designed for ease of use, allowing quick access to vital information and efficient management of train assets.
Figure 3. Bogie Health Asset Analysis View
The “Asset Analysis – Bogie” view offers a detailed examination of the bogie condition for a specific train. Key metrics such as spring compression, bearing temperature, bearing vibration amplitude, and wheel vibration amplitude are displayed at the top, providing real-time data and thresholds for quick assessment. The central section features a visual representation of the bogie, highlighting specific issues such as exceeded health thresholds, with precise identification and error codes for easy response. To the right, the remaining useful life (RUL) prediction graph compares actual versus predicted RUL. Below, the running status of the bogie, including its train type, make, and description, is detailed for comprehensive monitoring.
Key Features:
- Comprehensive Bogie Health Metrics: This section displays vital health indicators for Bogie B001, including vibration levels, temperature readings, and overall condition assessments. It contrasts real-time health data with predictive analytics to forecast the remaining useful life of the bogie, enhancing maintenance planning.
- Interactive 2D and 3D Bogie Models: The view features detailed 2D and 3D models of Bogie B001, offering capabilities to ‘explode’ the view for a closer examination of individual components. Critical areas, flagged by predictive analysis for potential wear or failure, are highlighted in the model for quick identification. For example, areas showing abnormal wear patterns are marked in distinct colors.
- Error Identification and Proactive Recommendations: Users can interact with highlighted areas on the bogie model to access specific error details and associated recommendations. This direct linkage to XMPro’s Recommendation Manager facilitates swift and effective resolution strategies.
- Detailed Bogie Information: The dashboard provides extensive information about Bogie B001, including its type, model, operational history, and manufacturer details, offering a complete profile of the asset.
- XMPro Co-Pilot Integration: Integrated with XMPro Co-Pilot, this feature utilizes AI, trained on internal datasets like maintenance records and manufacturer specifications, to provide targeted advice and solutions for issues related to Bogie B001. This AI-driven assistance supports informed decision-making and enhances the efficiency of maintenance processes.
This Asset Analysis View is tailored to deliver a comprehensive understanding of Bogie B001’s health, combining advanced visualizations with data-driven insights and AI-powered recommendations for effective bogie management in the rail industry.
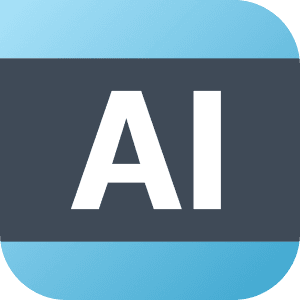
XMPro AI
Experience the transformative power of XMPro’s Intelligent Business Operations Suite (iBOS) – Featuring comprehensive AI capabilities, XMPro iBOS helps to significantly increase product yield, drastically reduce downtime, and ultimately eliminate unexpected business events.
Figure 1: Embedded AI Example – Bogie Health
Embedding XMPro AI Agents in XMPro Data Streams enables executable AI and machine learning for algorithmic business processes, significantly enhancing the capabilities of operational digital twins. This integration allows for advanced features such as real-time analytics, MLOps, and seamless embedding of AI into core business processes.
In the example of bogie health condition monitoring, XMPro’s AI Agents empower the data stream to accurately identify and predict potential bogie issues. The process begins with the ingestion of real-time sensor data, including wheel vibration, bearing vibration, bearing temperature, and spring compression, combined with historical work orders and past unplanned downtime data. The data is normalized and contextualized with bogie ID and operational information from digital twins.
Machine learning models, including anomaly detection and remaining useful life (RUL) prediction, are applied to detect deviations and forecast maintenance needs. The results are filtered, merged, and broadcasted for further action. The data stream generates actionable insights for maintenance scheduling and resource allocation, ensuring optimal bogie performance and minimizing the risk of failures.
Embedded AI Agents
XMPro offers a variety of AI agents to support diverse operational needs, including:
- Azure OpenAI: Enhances natural language processing capabilities.
- OpenAI Assistant: Facilitates conversational AI integrations.
- Anomaly Detection: Identifies unusual patterns in data to prevent operational failures.
- Forecasting: Predicts future trends based on historical data.
- Kmeans Clustering: Groups similar data points for more effective analysis.
- MLflow: Manages the machine learning lifecycle, including experimentation, reproducibility, and deployment.
- Regression: Provides predictive analytics to understand relationships between variables.
By embedding these powerful AI agents, XMPro transforms AI models into valuable assets that drive business growth and efficiency, bridging the gap between data flow and operational AI.
Why XMPro iBOS Bogie Health Condition Monitoring In The Rail Industry?
XMPro’s Intelligent Business Operations Suite (iBOS) is uniquely equipped to address the complexities of Bogie Health Monitoring in the rail industry, utilizing cutting-edge technology and analytics. Here’s how XMPro iBOS excels in this application:
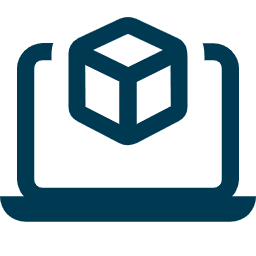
Intelligent Digital Twin for Bogie Monitoring:
XMPro iBOS allows users to craft an intelligent digital twin of the bogie components, enabling detailed simulation and analysis. This virtual model is crucial for assessing wear patterns and predicting maintenance needs, enhancing the accuracy of anomaly detection.
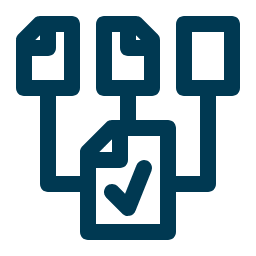
Seamless Data Integration and Transformation:
Featuring a robust integration library, XMPro iBOS seamlessly incorporates data from various sensors, including vibration and temperature sensors on bogies. This integration is key to transforming raw data into meaningful insights for predictive maintenance.
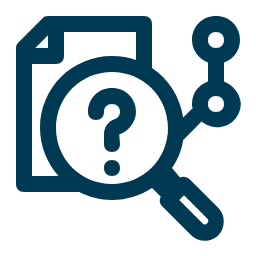
Advanced Machine Learning for Anomaly Detection:
Utilizing machine learning algorithms, XMPro iBOS analyzes sensor data to identify anomalies that indicate abnormal wear. This approach allows for early detection of potential issues that could lead to derailments or other safety hazards.
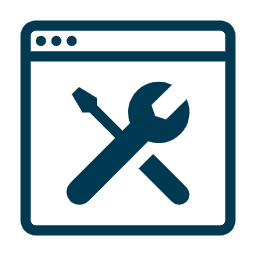
Optimization of Maintenance Schedules:
By analyzing wear patterns and predicting maintenance needs, XMPro iBOS helps optimize maintenance schedules. This shift from fixed-interval to condition-based maintenance reduces costs and prevents unnecessary downtime.
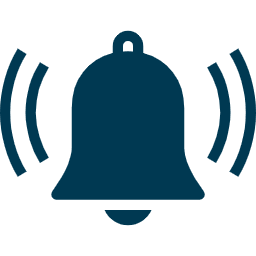
Real-Time Alerts and Proactive Decision Making:
The system provides instant alerts on emerging bogie issues, coupled with decision support tools. This feature aids maintenance teams in prioritizing actions based on the criticality of the detected anomalies.
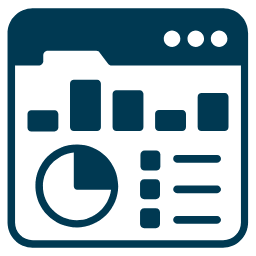
Customizable Dashboards for Enhanced Visibility:
XMPro iBOS includes adaptable dashboards that display vital bogie health data, alongside comprehensive reporting features for maintenance planning and compliance purposes.
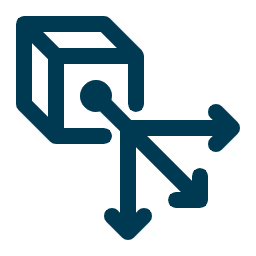
Scalability and Flexibility – Start Small, Scale Fast:
Designed for scalability and flexibility, XMPro iBOS can be tailored to various rail network sizes and integrates effortlessly with diverse sensor technologies. Its rapid deployment capability ensures a quick realization of value.
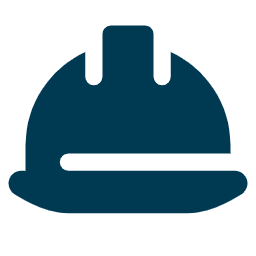
Enhanced Safety and Operational Efficiency:
By enabling proactive maintenance and early wear detection, XMPro iBOS significantly enhances the safety and operational efficiency of rail systems, reducing accident risks and ensuring reliable service.
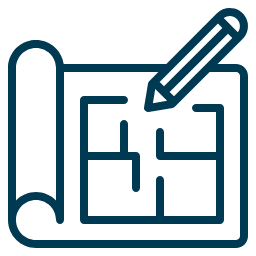
Quick Time To Value – XMPro Blueprints
Utilize XMPro blueprints, pre-configured for bogie health monitoring, to quickly set up the digital twin dashboard. These blueprints integrate industry best practices, ensuring a swift and effective implementation.
In essence, XMPro iBOS offers a holistic, real-time, predictive, and integrated approach to bogie health monitoring. Its advanced capabilities in digital twin modeling, data integration, machine learning, and configurable dashboards make it an invaluable asset for ensuring the safety and efficiency of rail operations.
Use XMPro Blueprints for Quick Time To Value
Easily import Blueprints, Accelerators and Patterns into your environment, providing a starting point for configuring your own solutions.
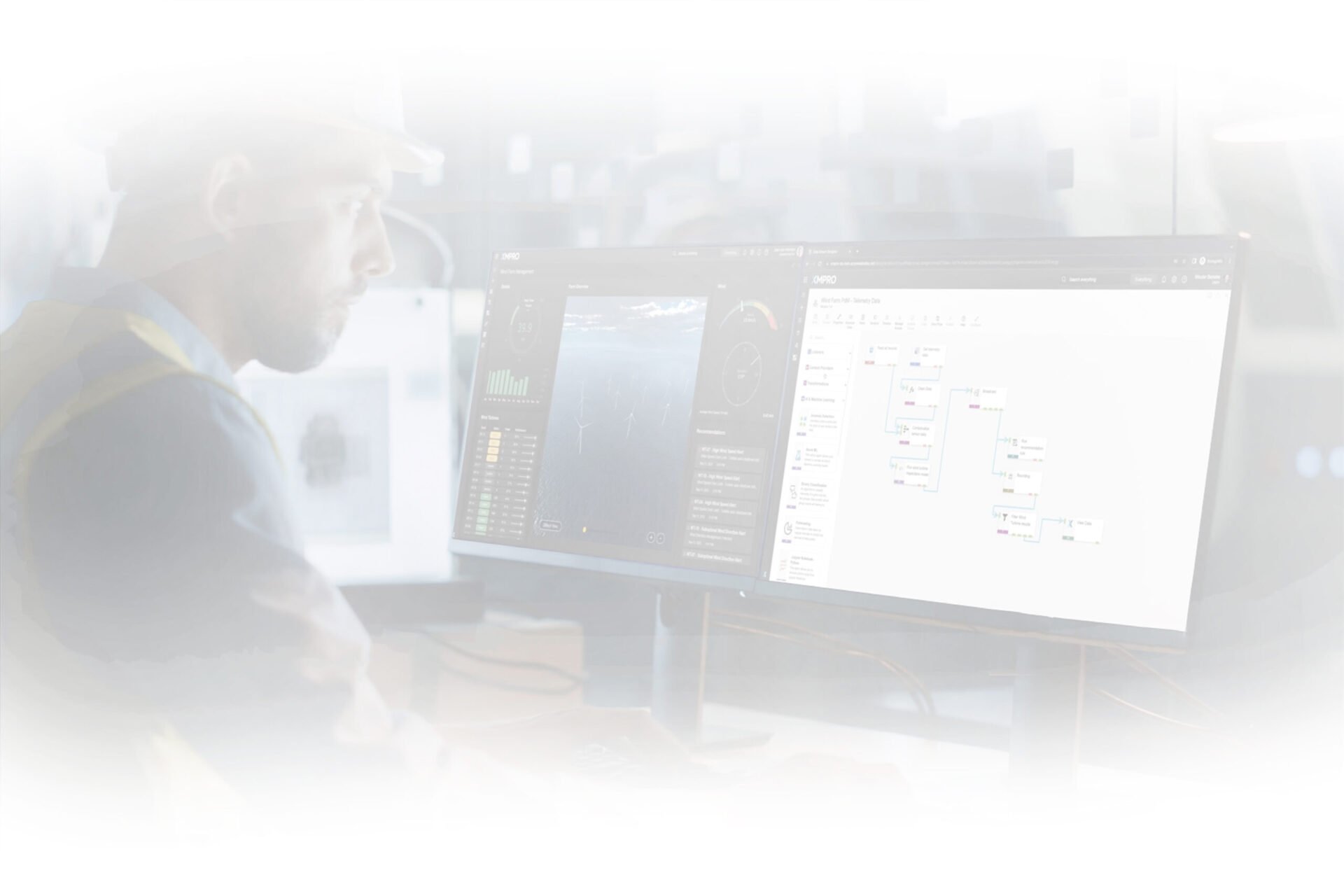